By: April Carson
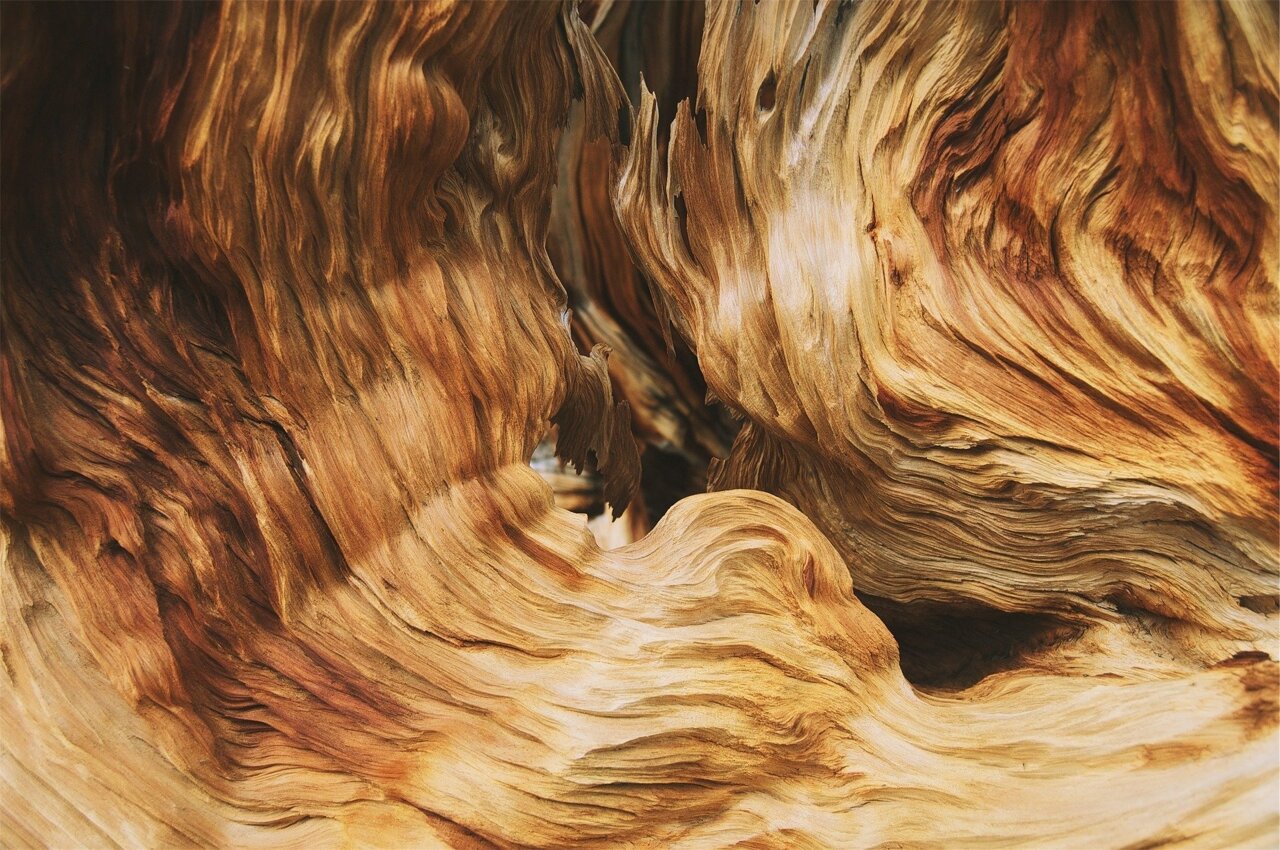
The increasing use of 3D printing for manufacturing a wide range of goods, from customized medical devices to low-cost houses, has increased demand for new 3D printing materials tailored to particular functions.
Researchers at MIT have created a data-driven procedure that employs machine learning to optimize new 3D printing materials with varying properties, such as toughness and compression strength, in order to save time.
By streamlining material production, the technology lowers expenses and minimizes environmental impact by lowering chemical waste. The machine learning algorithm might also lead to innovation by suggesting unique chemical formulations that a person's intuition may miss.
"Materials development is still largely a manual process. A chemist enters a lab, combines chemicals by hand, obtains samples, and runs tests before reaching a conclusion. But our method may do hundreds of iterations in the same period as opposed to just a few over several days for a chemist," says Mike Foshey, co-lead author of the paper and principal materials scientist at the Naval Research Laboratory (NRL).
In the system the researchers developed, an optimization algorithm performs much of the trial-and-error discovery process. In an optimization problem, the goal is to maximize or minimize a value—as defined by a mathematical function—subject to certain constraints. In this case, the task was to find a combination of chemicals that yield a material with a high index-of-refraction and low photoluminescence lifetime.
A material developer picks a few components, enters chemical information into the algorithm, and establishes the mechanical properties of the new material. The algorithm then raises and lowers the amounts of those components (as with a volume knob on an amplifier), testing each formula's impact on the material's qualities, before coming up with the best combination.
The developer then combines, processes, and tests the sample to see how well it performs. The results are shared with the algorithm, which learns from the study and adjusts future formulations based on the findings.
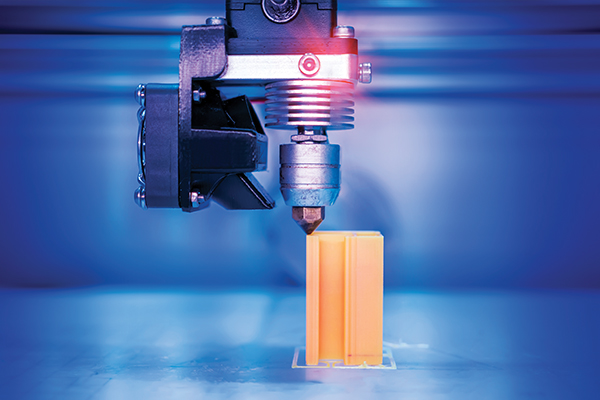
"We believe that this method would outperform the standard technique for a variety of applications because the optimization algorithm may be trusted to find the best answer more often. You wouldn't require a chemist with years of expertise to preselect material formulations," Foshey explains.
The scientists have established a free, open-source materials optimization platform called AutoOED that uses the same optimization algorithm. AutoOED is a comprehensive software package that also allows researchers to optimize their own data.
Making materials
The system was tested by optimizing formulations for a new 3D printing ink that hardens when exposed to UV light.
They identified six chemicals to use in the formulations, and the algorithm's objective was set to find the best-performing material with respect to hardness, compression modulus (stiffness), and strength.
The three properties that must be optimized manually are strength, durability, and flexibility. These qualities can be in conflict; for example, a strong material might not be the stiffest. A chemist would typically attempt to improve one property at a time using a manual method, resulting in many test runs and waste.
After evaluating only 120 samples, the algorithm generated 12 top performing materials that offered optimal tradeoffs in terms of all three qualities.
De Deo et al. were shocked by the wide range of materials that the algorithm was able to produce, and they remark that the results were far more diverse than anticipated based on the six components. The method encourages research, which might be especially beneficial in situations when particular material properties cannot be immediately recognized intuitively.
In the future, it will be faster
The use of automation might even speed things up even more. Researchers hand-mixed and tested each sample, but in future versions of the system, robots could run the dispensing and combining equipment.
The researchers would also like to apply their data-driven discovery process to other applications in the future, such as designing new 3D printing ink.
"This has significant implications beyond materials science. For example, if you wanted to create new types of batteries that were more efficient and less expensive, you could use a method like this to do it. This system might also be used to optimize paint for a vehicle that performs well while being ecologically responsible," he adds.
4biddenknowledge Podcast: Elevated Insights With Kxng Crooked By Billy Carson
---------------
About the Blogger:
April Carson is the daughter of Billy Carson. She received her bachelor's degree in Social Sciences from Jacksonville University, where she was also on the Women's Basketball team. She now has a successful clothing company that specializes in organic baby clothes and other items. Take a look at their most popular fall fashions on bossbabymav.com
To read more of April's blogs, check out her website! She publishes new blogs on a daily basis, including the most helpful mommy advice and baby care tips! Follow on IG @bossbabymav
-------------------
LOOKING TO INVEST IN 4BIDDENKNOWLEDGE? CLICK HERE TO MAKE A CONTRIBUTION AND GET INVOLVED WITH 4BIDDENKNOWLEDGE TV AND BECOME PART OF THE MOVEMENT!
CLICK THE LINK TO INVEST